1 |
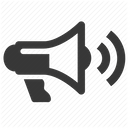 |
Course introduction. Housekeeping What is computational economics. Course structure and house keeping. Choice of programming language. |
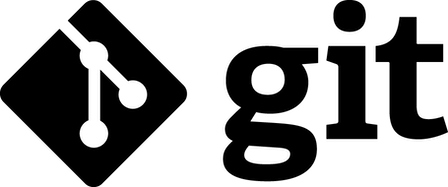 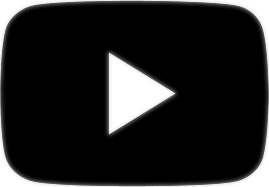  |
2 |
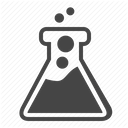 |
Local workspace. Jupyter notebooks. Git and GitHub Introduction of Git version control system. Local installation of Python, Anaconda, Jupyter Notebooks, Git and Git GUI. GitHub and GitHub Classroom. |
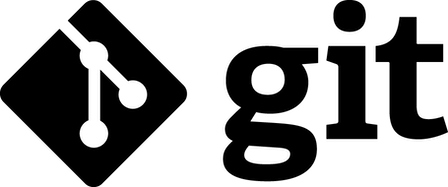 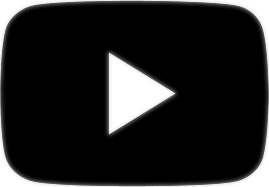  |
3 |
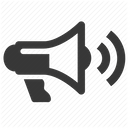 |
Representing numbers in a computer Binary and hexadecimal numbers. Floating point numbers. Numerical stability and potential issues. Numerical noise. |
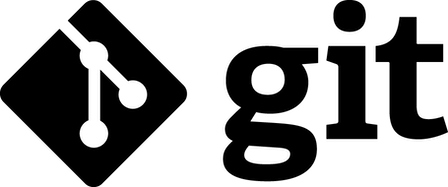 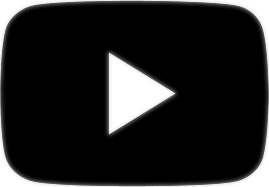  |
4 |
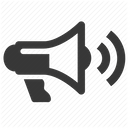 |
Python essentials: data types Variables and memory, binary operations, logical expressions, composite variables types. |
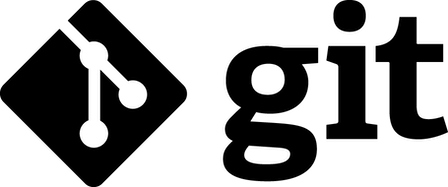 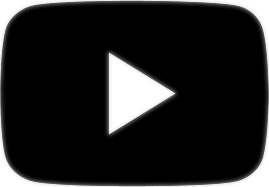  |
5 |
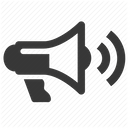 |
Python essentials: control flow and functions Flow control, user defined functions. Sieve of Eratosthenes example. |
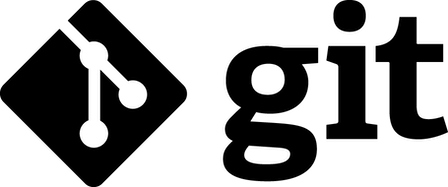 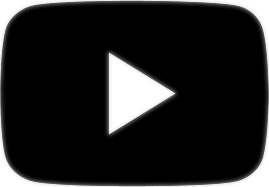  |
6 |
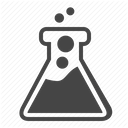 |
Two simple examples Indexing problem and its inverse, base-N number conversion |
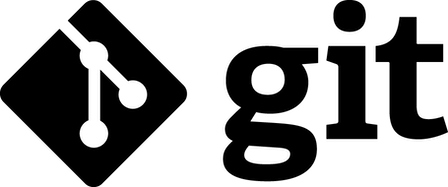 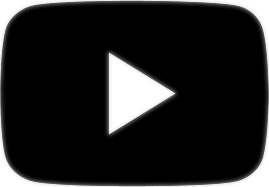  |
7 |
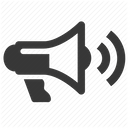 |
Python essentials: object-oriented programming Classes and objects. Attributes, properties. Encapsulation, inheritance and polymorphism. |
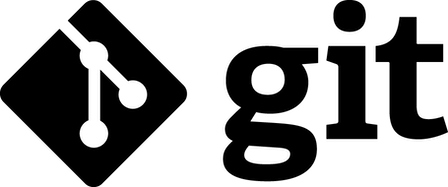 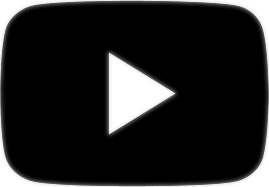  |
8 |
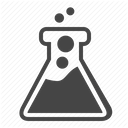 |
Bundle goods market Object oriented programming in modeling consumer choice model. |
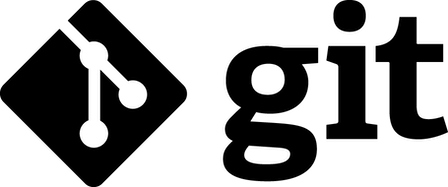 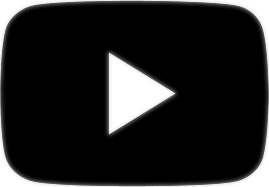  |
9 |
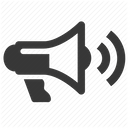 |
Algorithms and complexity Timing of Python code. Runtime order of growth. Complexity classes. P vs NP. |
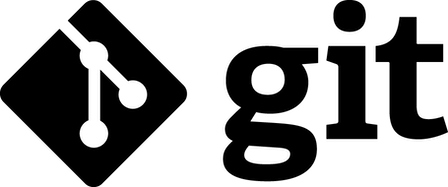 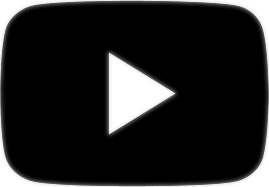  |
10 |
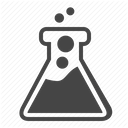 |
Two simple algorithms: parity and max Parity of a number, bitwise operations in Python. Finding maximum in an array. |
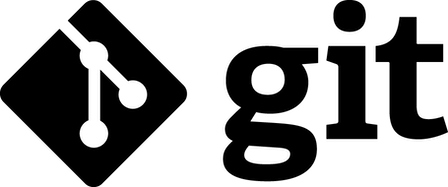 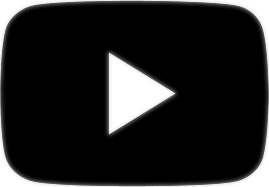  |
11 |
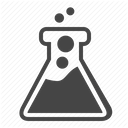 |
Binary search algorithm Binary search. Other divide and conquer algorithms. Recursion. |
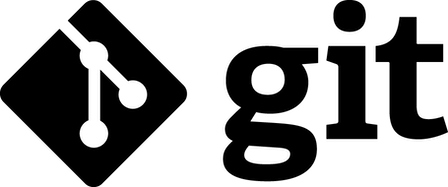 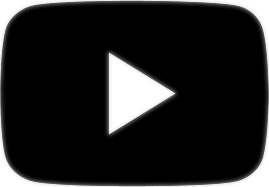  |
12 |
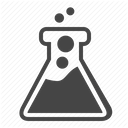 |
Enumeration of discrete compositions Combinatorial enumeration. Python generators. |
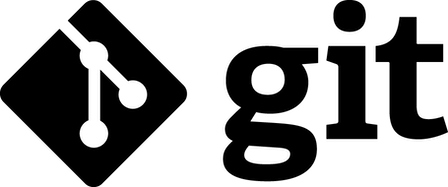 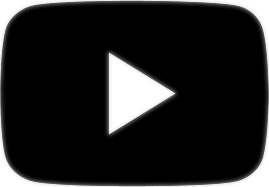  |
13 |
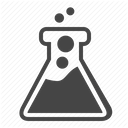 |
Two very important algorithms for solving equations Bisections and Newton-Raphson methods. Solving equations of one variable. Accuracy of solution. Rates of convergence. |
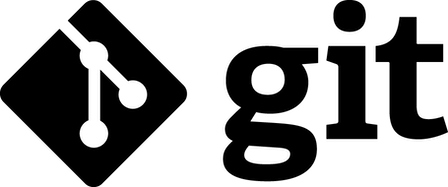 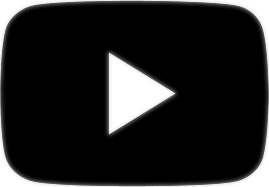  |
14 |
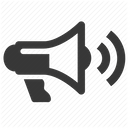 |
Vectors and matrixes (Numpy) NumPy arrays data types and differences to native Python, operations on the arrays, solving linear systems of equations. |
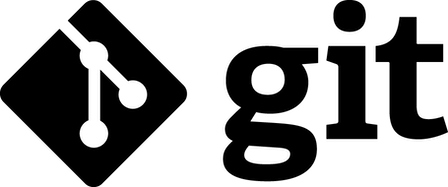 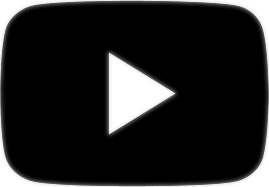  |
15 |
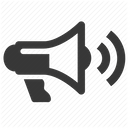 |
Introduction to Data Manipulation in Python (Pandas) Introduction into DataFrames, grouping and data merging. |
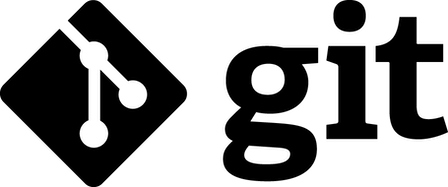 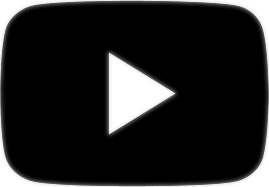  |
16 |
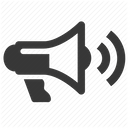 |
Visualization of data and solutions Principles and functions of graphics. Examples of visualization of economic models. |
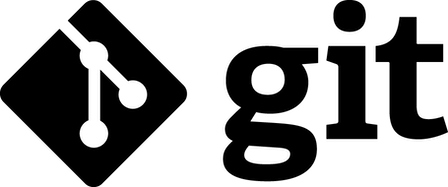 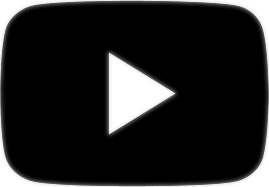  |
17 |
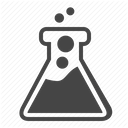 |
Linear regression using Pandas and Numpy Using Numpy and Pandas to estimate simple regression. |
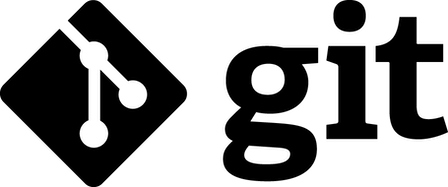 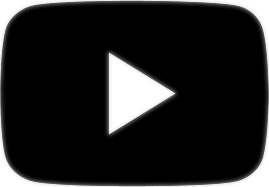  |
18 |
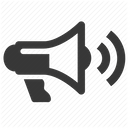 |
Linear programming and optimal transport models Linear programming and optimal transport problems. |
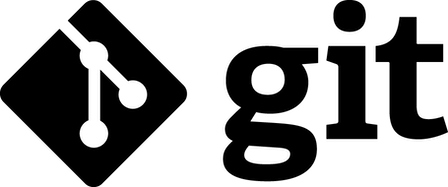 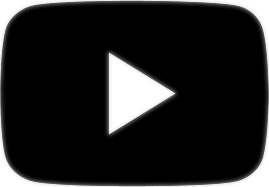  |
19 |
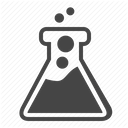 |
Measuring the volume of illegal trade with linear programming Application of the optional transport problem. |
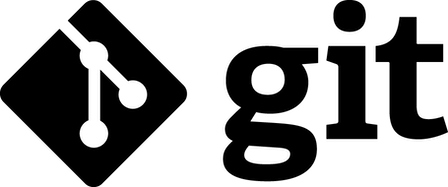 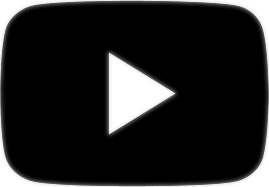  |
20 |
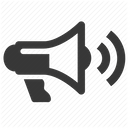 |
Finite Markov chains Stochastic matrix, irreducibility and aperiodicity, stationary distribution. |
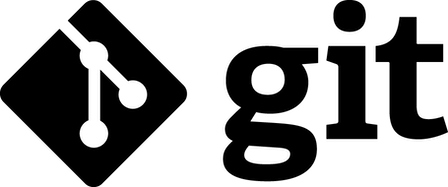 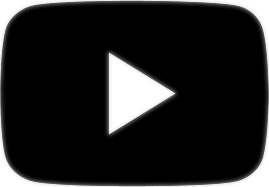  |
21 |
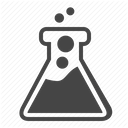 |
Computing a stationary distribution of a Markov chain Successive approximations and direct linear solver. |
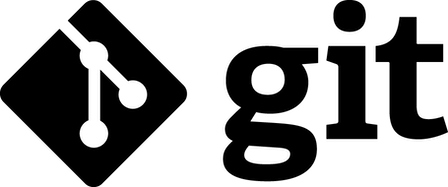 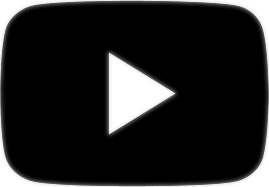  |
22 |
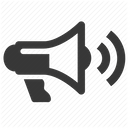 |
Successive approximations (fixed point iterations) Scalar and multivariate solver. Equilibrium in market of platforms. |
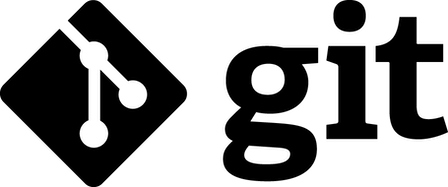 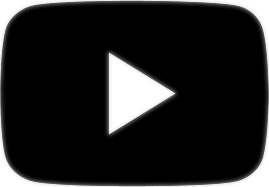  |
23 |
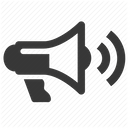 |
More on Newton-Raphson method Failures of Newton method, domain of attraction. Multivariate Newton for optimization problems. |
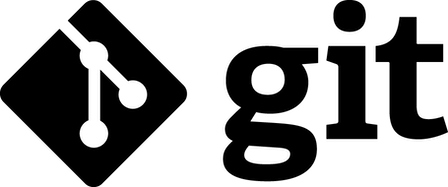 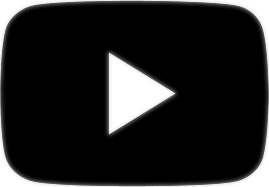  |
24 |
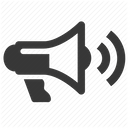 |
Optimization through discretization (grid search) Grid search method and its use cases. |
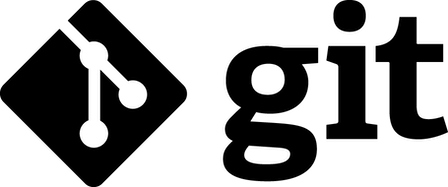 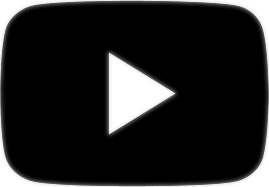  |
25 |
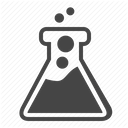 |
Newton-Raphson method with bounds Robust implementation of Newton method for problems with strict bounds. |
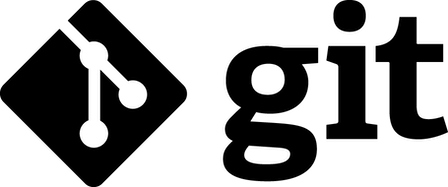 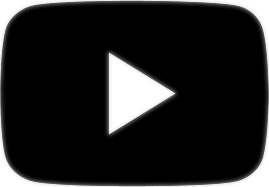  |
26 |
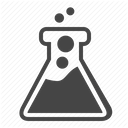 |
Polyline class for piecewise linear curve approximation Precomputation of complex curves in the equilibrium model (coding from scratch). |
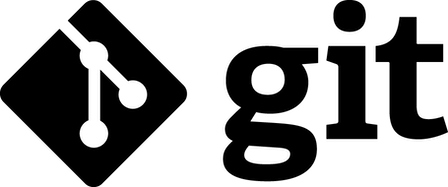 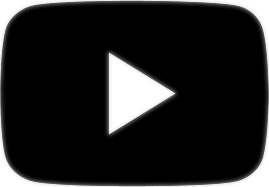  |
27 |
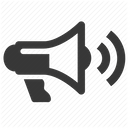 |
Dynamic programming in discrete world Backwards induction. Tiling problem. Deal or no deal game. Bellman optimality principle. Inventory dynamics model. |
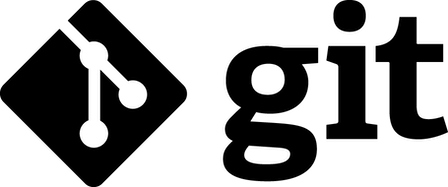 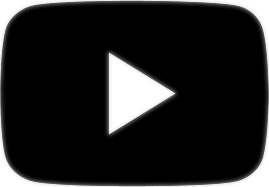  |
28 |
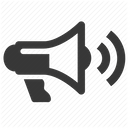 |
Rust model of bus engine replacement Model background and formulation. Mileage process. Optimal replacement choice with and without EV taste shocks. |
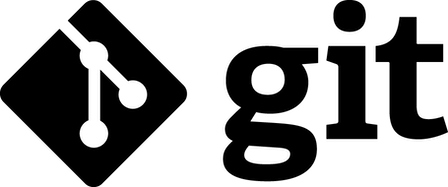 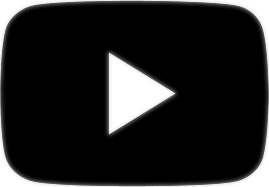  |
29 |
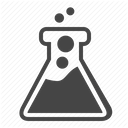 |
Coding up the Rust model of bus engine replacement Implementation of Rust model in infinite horizon with value function iterations solver |
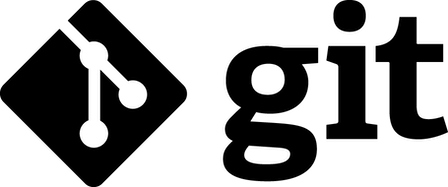 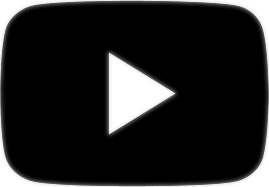  |
30 |
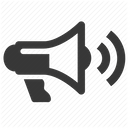 |
Cake eating in discrete world Cake eating problem setup. Solution “on the grid”. |
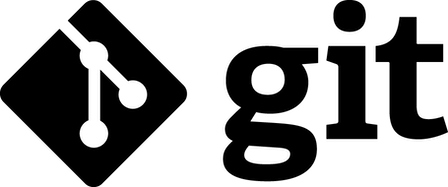 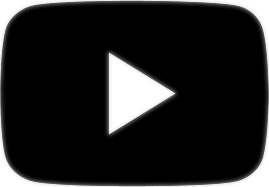  |
31 |
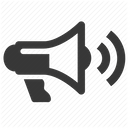 |
Function approximation in Python How to approximate functions which are only defined on grid of points. Spline and polynomial interpolation. |
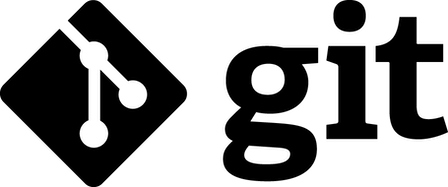 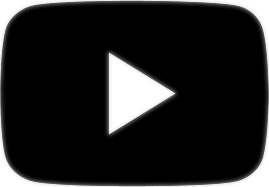  |
32 |
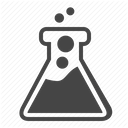 |
Cake eating model with discretized choice Using function interpolation to solve cake eating problem with discretized choice. |
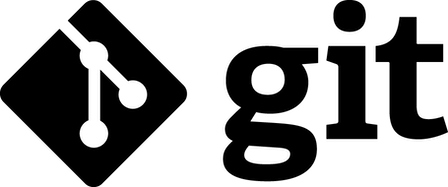 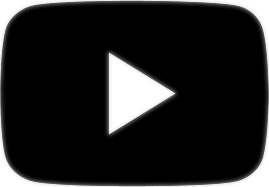  |
33 |
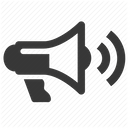 |
Random numbers in Python, Monte Carlo Random number generation in Python. Inverse transform sampling. Monte Carlo simulations. |
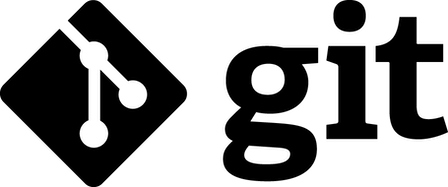 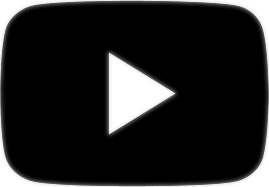  |
34 |
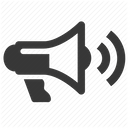 |
Numerical integration, quadrature Gaussian quadrature. Monte Carlo integration. |
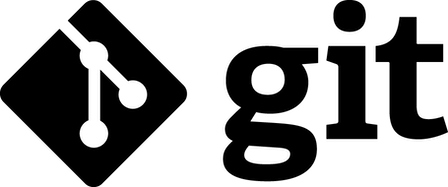 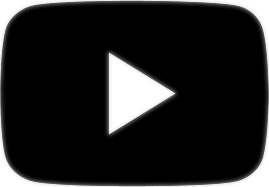  |
35 |
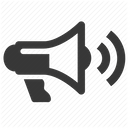 |
Stochastic consumption-savings model with discretized choice Deaton model of consumption and savings with random returns. Using quadrature to compute the expectation in the Bellman equation. |
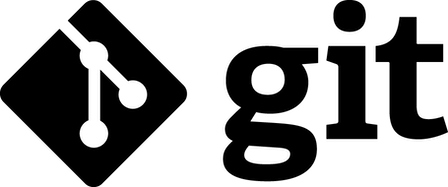 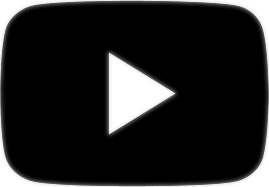  |
36 |
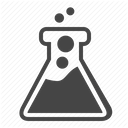 |
Simulating data from the model Random variables induced by the model. Coin flipping example. Simulating consumption and wealth paths from the consumption-savings model. |
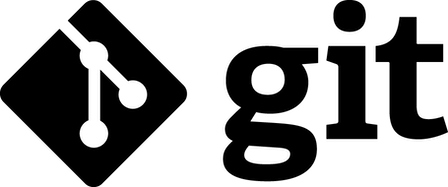 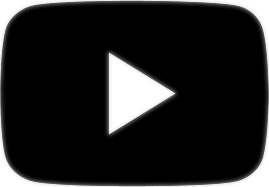  |
37 |
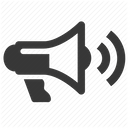 |
Dynamic programming theory and overview of solution methods Overview of dynamic programming problem formulations and solution methods. Theoretical foundations of dynamic programming in infinite horizon. Contraction mappings and fixed points. |
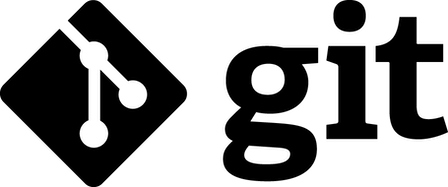 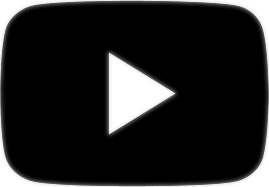  |
38 |
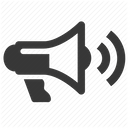 |
Dynamic programming with continuous choice Optimization in Python. Consumption-savings model with continuous choice. |
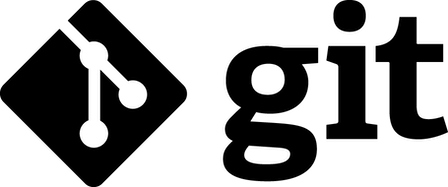 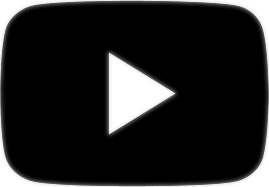  |
39 |
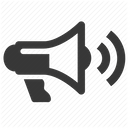 |
Euler equation and time iterations First order conditions and Euler equation. Time iterations solution method. Euler residuals for measuring the accuracy of solution for consumption-savings model. |
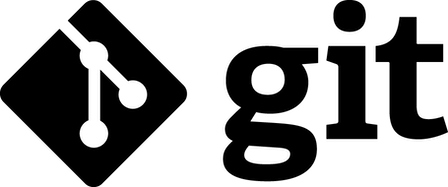 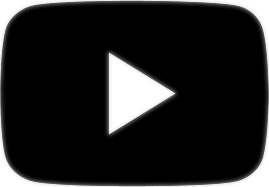  |
40 |
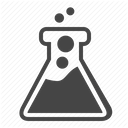 |
Consumption-savings model with continuous choice Adding continuous version of Bellman operator and time iterations solver to the consumption-savings model. Measuring accuracy of different solutions. |
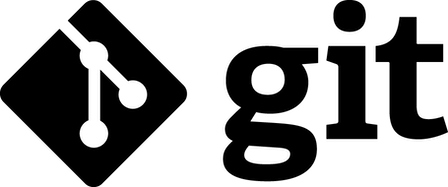 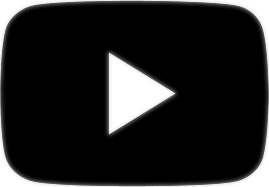  |
41 |
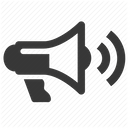 |
Endogenous gridpoint method (EGM) Fastest and most accurate solution methods for consumption-savings model. Class of models solvable by EGM. Generalizations of EGM method. |
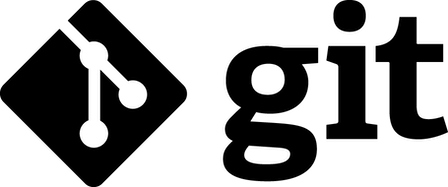 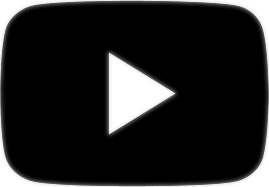  |
42 |
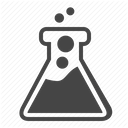 |
Solving consumption-savings model with EGM Implementation of endogenous gridpoint method for solving Deaton’s consumption-savings model. |
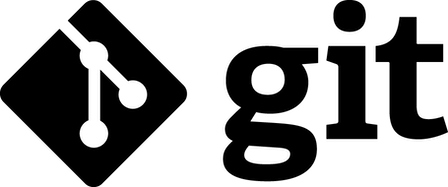 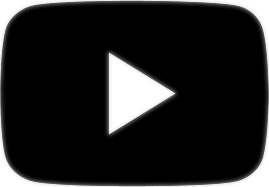  |
43 |
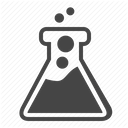 |
Solving DP problems with policy iterations Policy iterations solution method for infinite horizon dynamic models. Solving stochastic inventory management problem with policy iterations. |
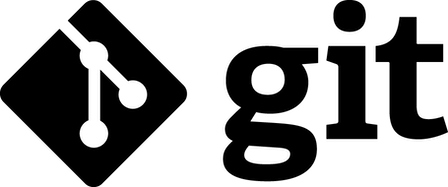 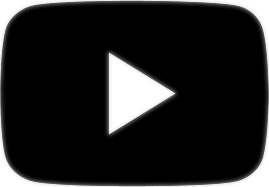  |
44 |
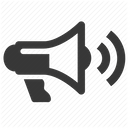 |
Newton-Kantorovich method Solving Bellman equation using Newton-Kantorovich iterations. Convergence rates. Polyalgorithm. |
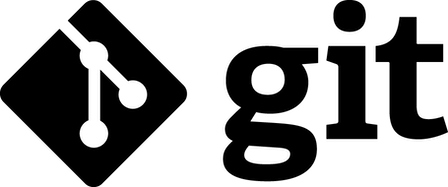 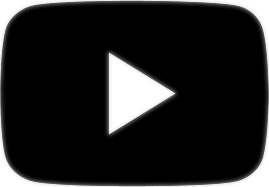  |
45 |
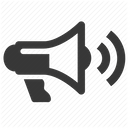 |
Method of simulated moments for model estimation (MSM) Using data to inform numerical economic models. Calibration and estimation of economic models. Introduction to method of simulated moments (MSM). |
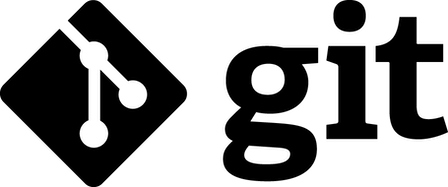 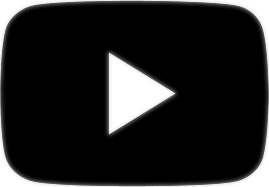  |
46 |
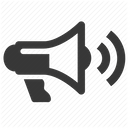 |
Nested fixed point maximum likelihood estimator (NFXP) Nested loop MLE estimator. Combining Newton-Kantorovich iterations with gradient based likelihood maximization. Structural estimation of Rust bus engine replacement model. |
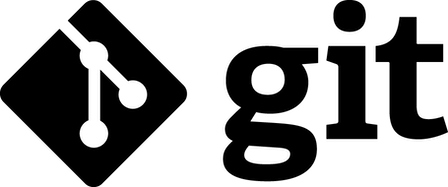 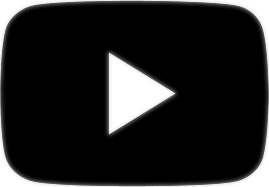  |
47 |
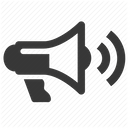 |
Example exam questions Examples of questions and answers in the exam. |
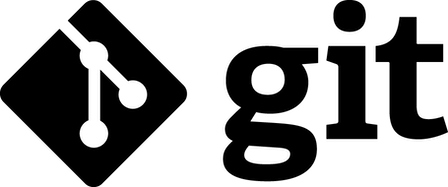 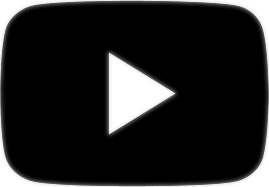  |